Any customer interaction generates a wealth of data, hidden inside of which are vital insights into improving the customer experience. That data is chiefly in the form of speech and text, so revealing those insights requires an analytics solution that can trawl through the increasingly vast amounts collected and separate the wheat from the chaff.
In this edition of the CX Today round table, we welcome:
- Sharon Einstein, Vice president and general manager, NICE Experience Analytics
- Dan O’Connell, CSO, Dialpad
- Paul Lang, Senior Director, Contact Centre Solutions Marketing, Avaya
- Frank Sherlock, VP of International, CallMiner
Our panellists will discuss the major hurdles for businesses wanting to adopt speech and text analytics, how the market is developing, and how the deployment of machine learning has impacted growth.
What are the major hurdles for businesses wanting to adopt speech and text analytics as we move into 2022?
Einstein: Analytics adoption is becoming widespread in contact centres, while at the same time businesses are prioritising customer satisfaction over more traditional contact centre metrics. Still, some organisations are not taking advantage of the recent advances in speech and text analytics, which can effectively advance customer experience strategies. The main barrier to implementation continues to be for those businesses that persist in using legacy premise-based IT solutions.
Many businesses continue to have contact centres that function in silos. The centres themselves are generating plenty of data, but there’s no systematic approach to collect that data into a single source of truth from which analytics experts can analyse and devise actionable insights. Advanced speech and text analytics are only really achievable with a single, integrated cloud platform that is pulling data from across the contact centre ecosystem.
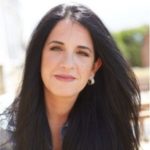
O’Connell: Voice data is the last offline dataset. So much data is lost in voice calls, whether that’s group meetings, one-to-ones, outbound sales conversations and inbound service calls. You end up repeating meetings to get to decisions, forgetting actions or decisions that were made, or missing out on valuable customer insights. Even with all the values of speech and text analytics, businesses are still slow or hesitant to adopt.
Providing a clear, efficient and cost-effective solution to this problem is critical to proving the value of speech and text analysis to businesses. Even once businesses realise the value of voice, cost can be another hurdle.
We’ve found that technology stacks built internally instead of using other platforms prove to be much more cost-effective. Our technology has been built entirely in-house instead of relying on other outsourced products so we’re able to control more and drive costs down to give our customers the most value.
Accessing the insights hidden in voice interactions can lead to huge cost savings, increased sales, time savings, and improved productivity, so it can affect every corner of the business and impact the top line and bottom line simultaneously.
Lang: One of the biggest challenges in customer services is being able to collect, collate, analyse, interpret tons of data in real time and do something meaningful with it. One thing that can make a big difference is conversational AI which can process words and voice conversations in real time then respond with contextual information, unlike traditional speech analytics applications that mine calls after they occur.
Conversational AI incorporates technologies like chatbots or voice assistants, with machine learning to improve customer interactions. Research shows the most successful companies are moving cautiously with AI, starting with agent assist or chatbots (nearly 40% of companies are leveraging AI-powered chatbots to interact with customers). Although many companies are not considering conversational AI, today’s biggest brands, such as Facebook, Apple, and Google, are signalling AI’s rise by making key investments in conversational design.
Conversational AI is something organisations are going to have to contend with for future success in customer experience.
Sherlock: A key hurdle that contact centre leaders will continue to face in the coming year is getting executive buy-in to invest in speech and text analytics.
While contact centre leaders are well versed on the benefits of conversation analytics, such as automated QA, improved agent performance, increased customer satisfaction and more, the budget for these solutions often falls outside of the contact centre, such as with customer experience executives or even at the C-suite level. Getting them to allocate budget isn’t always easy, until they understand how the solutions can deliver ROI and impact the bottom line.
Another hurdle is getting internal teams to embrace the solutions, especially the agents who interact with the technology on a daily basis. It’s important to explain and show the people engaging with these tools most frequently how the insights that are uncovered are to their benefit. It’s not about monitoring them – it’s about helping to support them in their roles, making their jobs easier and giving them access to the objective, data-driven feedback it takes to improve.
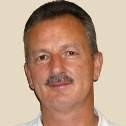
How will the speech and text analytics market develop over the next year and what will this mean for businesses and their customers?
Einstein: Speech and text analytics are an indispensable part of the modern contact centre’s toolkit. Text and voice interactions comprise the largest percentage of unstructured data in most contact centres in the UK. Making sense of all the data being generated about a company – from social-media channels, chats with customer-service agents, surveys, forms, warranty claims, and more – is going to become a key differentiator for businesses.
Advanced analytics allow companies to conduct end-to-end analytics on millions of customer data points to proactively identify potential improvements. Initially, such benefits may be incremental in some areas of the business while in others it may be more noticeable. However, what is clear is that those who start applying these tools now and begin learning through the experience will reap the rewards down the line. Having clean, usable data is akin to the idea of compound interest, it’s continually built upon with long-term benefit.
O’Connell: Beyond the practical applications of call transcription, real-time coaching and sentiment analysis, voice data is rich with data and can be leveraged like any other data set- and it will only get smarter over time.
Once voice becomes a company’s data set, that data can become used like any other set – you can get instant access to the number of times a word is mentioned and then trigger technology in other parts of the business to action (ie. If there is a large increase in customers saying the word “refund”).
Being able to dig into the analytics of customer satisfaction, for example, is a game-changer. With embedded AI, we can think about how to automate certain workflows which is where additional gains will be made. We really are in the early innings of AI, but we can definitely picture a future where users can say they need to reschedule a meeting during a call and the calendar immediately finds the meeting participants’ availability and schedules the time, or a future where technology has evolved to the point where sales teams no longer have to input data into a CRM, but instead, the data could be auto-populated.
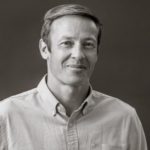
Lang: Conversational AI is the next big development as it helps deliver deeper and more personalised customer engagements – for example: when recognising certain words in a conversation between agent and customer, a screen automatically pops up that displays step-by-step directions for the product that customer has just bought and is having trouble using.
By infusing conversational AI, contextual knowledge, and insights throughout every customer journey, businesses can enable more intelligent decisions and informed next best actions. This benefits not just the customers but also agents as it transforms unstructured phone conversations into actionable intelligence, which can be used to improve performance, call handling, first call resolution, self-help content, and more. It can also be shared with product development, back-office operations, sales, marketing, and other departments to drive transformation into a customer-led business.
After-call work can also be reduced as conversational AI automates post-call reporting and other form-fills, like CRM inputs, to help agents better serve customers and move immediately to the next customer. The technology significantly reduces (if not eliminates) error-prone, incomplete, and time-consuming manual tasks.
Sherlock: The benefits of speech and text analytics have primarily been experienced by the contact centre, but the value that exists within customer conversations has the potential to benefit departments across organisations. Over the next year, brands will stop keeping that data – and the insights held within those conversations – siloed in the contact centre.
For example, customers often call contact centres and have conversations that involve feedback on products, such as difficulty finding a certain product in store, the most common complaints that result in warranty claims and more. This type of feedback should be routed directly to product development leaders. They can up production of a certain product to meet demand needs or rework pipeline development to prioritise updates that address product flaws – or even to develop new products all together.
The companies who use the insights that are uncovered in customer conversations to drive improvements enterprise-wide will be the ones who will rise above the competition in the coming year.
How has the deployment of machine learning impacted the growth of the speech and text analytics market?
Einstein: The deployment of machine learning and AI is a game-changer in the analytics market with those businesses taking advantage of it seeing the biggest return on investment. Near-instantaneous analysis of unstructured – predominantly voice – data is already allowing real-time agent guidance on customer interactions. For instance, speech analytics can recognise a client’s hostile tone in reaction to an agent’s poorly phrased question and guide them with clear written and visual prompts to rephrase and get the conversation back on track.
Customers’ demand for self-service is growing the need for analytics across digital channels. To make self-service work, machine learning is critical for businesses to learn from agent-assisted interactions how to improve experiences with virtual agents and chatbots. AI-driven self-service accelerates a business’ ability to continuously improve and respond to customers’ needs in the new digital world.
O’Connell: As an industry, we’ve only recently begun to leverage all the data that is typically lost in voice calls. For example, with ASR and NLP we’ve gotten to the point where manual note-taking and jotting down action items from calls are obsolete. The impact of ML on speech and text analytics has not only cut down on mundane daily tasks, but it’s also making the day a little easier. For example, instead of repeating meetings to get to decisions, or forgetting actions or decisions that were made during calls, we have call transcripts that cut down on follow-ups and give us valuable time back.
We’ve built our ASR and NLP technology from the ground up, which ultimately makes it more accurate. The focus is shifting from AI and machine-only automation like interactive voice response (IVR) and chatbots to harnessing AI’s power of real-time data collection and analysis so agents can operate with greater efficiency and provide hyper-personalised experiences for their customers.
The deployment of ML has allowed us to layer on AI so we can turbo-charge the benefits of our speech and text analytics, thus enabling continuous improvements and organisational efficiency, leading to better overall business performance.
Lang: Use of Machine Learning (ML) in speech and text analytics helps organisations more accurately, and speedily, mine enormous quantities of customer experience data of a varied nature. This data is a result of increased interaction volumes and numbers of communication channels.
ML in speech and text analytics has broadened and deepened the measurement of metrics beyond keywords to include tone, pitch, tempo and caller emotion. By analysing a combination of this information, ML-based solutions can offer more accurate insights into customer and employee experiences and other forms of business intelligence.
Currently available ML-based solutions not only analyse content from interactions but also add context and provide in-the-moment guidance for enhancing the customer experience, in real time. Using these capabilities, ML based applications assist by guiding agents through entire interactions.
AI and ML-infused speech and text analytics solutions are enabling businesses to track compliance, customer satisfaction levels, and product or service quality accurately and with greater speed. In this way, businesses can effectively better mine their contact centre’s customer experience data to win new customers, increase loyalty with existing customers, innovate new products and solutions and stay ahead of competitors, all of which drives revenue and customer satisfaction.
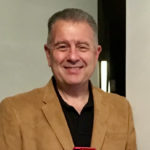
Sherlock: While there are still advancements to be made, artificial intelligence (AI) and machine learning (ML) make it possible to automate the interpretation of large and diverse stores of data. More information can be efficiently processed and transformed into valuable insights than ever before.
Beyond being able to analyse vast amounts of data, one of the most powerful outputs of AI and ML is the ability to impact contact centre interactions in real time. Speech and text analytics tools can recognise key conversation indicators, such as around customer emotion or compliance requirements. When those indicators are identified, such as a customer becoming increasingly irate during a conversation, AI-powered real-time features can deliver guidance to agents on how to handle those customer emotions or when it’s time to escalate to a supervisor. Similarly, if there are specific compliance statements that need to be said by an agent during a conversation, AI and ML can give agents reminders if they’ve missed those statements. This support increases compliance and reduces risk.
With AI and ML, speech and text analytics are delivering more value, from data analysis to agent support. These developments make it possible to efficiently drive improved business decisions, customer experience and more.